FinOps for AI: Managing Costs in the Age of Generative Intelligence
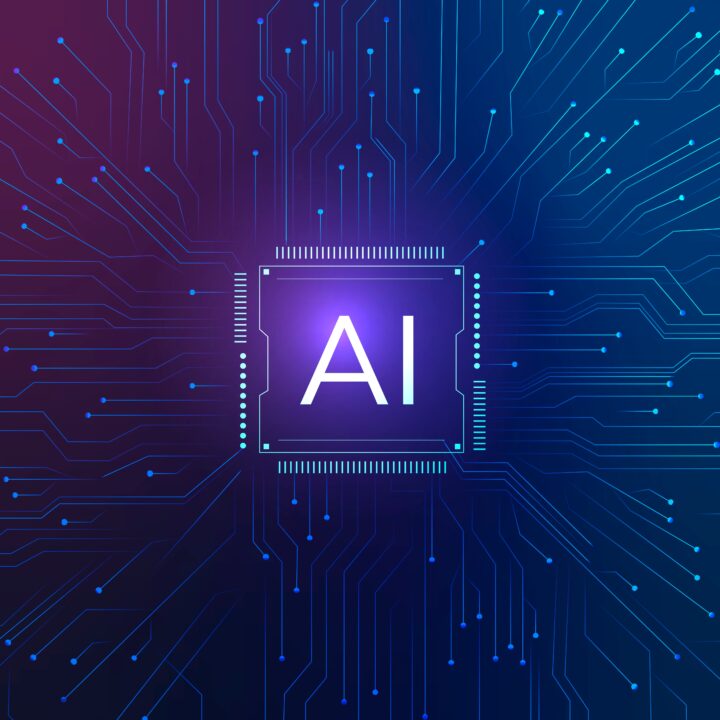
The FinOps Practitioner’s Definitive Guide To AI Services
FinOps for AI: Managing Costs in the Age of Generative Intelligence
I was excited to collaborate with Thavron Solutions on a joint whitepaper: The FinOps Practitioner’s Definitive Guide to AI Services. In it, Nan and I present a comprehensive roadmap for understanding AI cost structures, optimising spending, and ensuring that AI investments deliver measurable business value. Below, I share key insights from the paper and how FinOps professionals can take control of AI-related costs.
AI Growth Brings Financial Risk
By 2026, 75% of businesses will generate synthetic customer data with AI, yet more than half will abandon AI initiatives due to cost mismanagement (Gartner). The demand for AI services continues to soar, but without financial discipline, inefficiencies and uncontrolled spending can derail even the most promising projects.
For FinOps teams, this represents a major opportunity—and a major challenge. Financial oversight is essential to ensuring long-term viability of AI adoption and avoiding costly mistakes.
Understanding AI Cost Drivers
AI cost structures differ significantly from traditional IT services. FinOps teams must develop a granular understanding of the key cost components that drive total spend:
- Serverless AI Services – Offer flexibility and usage-based pricing, but often introduce unpredictability in monthly bills.
- Training Costs – Upfront training phases often account for 80–90% of total AI costs, especially for generative models.
- Infrastructure – Choosing between cloud or on-prem requires careful planning to balance performance and efficiency.
- Labour and Skills Gaps – AI talent is scarce and expensive. Outsourcing and upskilling must be evaluated carefully.
- Data Movement and Management – Storing, processing, and transferring training data can incur significant and often overlooked costs.
Expert Insights from Janine Pickard-Green
Janine Pickard-Green has spent the past decade in IT Finance, specializing in FinOps, IT Financial Management, and Cloud Cost Optimization. She has led cloud financial management solutions for top financial services and retail enterprises, focusing on cost allocation, budgeting, and forecasting. As an active member of the FinOps Foundation, Janine has contributed to the FinOps for AI working group, the FOCUS initiative, and cost allocation methodologies, helping organizations enhance financial visibility and accountability.
FinOps Principles for AI
Embedding FinOps practices early in AI adoption allows organisations to establish financial control before spending escalates. Key approaches include:
- Transparent Cost Modelling – Map AI costs to business outcomes and define ROI expectations from day one.
- Optimised Service Consumption – Apply usage controls and monitor real-time spend to avoid waste.
- Strategic Infrastructure Planning – Consider long-term needs and avoid short-sighted decisions that lock in cost.
- Governance and Risk Management – Ensure that security, compliance, and ethical considerations are included in cost assessments.
The Role of FinOps in AI Success
AI cost governance is no longer optional—it’s essential. From my experience as FinOps Lead at MagicOrange, a successful AI strategy depends on cross-functional collaboration and clear financial visibility. Here’s what I’ve learned:
- Track Generative AI Spend Early – Most of your total compute cost will occur during training, before the application ever reaches production. Understanding and managing this early spend is critical.
- Use Serverless Where Practical – Serverless services with usage-based pricing can significantly reduce cost exposure in the training phase, compared to fixed compute resources.
- Leverage Pre-Trained Models – Platforms like Hugging Face or vendors such as AI21 Labs offer robust pre-trained models. These are often more cost-effective than building from scratch.
- Start Small, Then Scale – Experiment with lightweight models and smaller datasets. This allows teams to refine training methods without committing excessive resources.
- Model Costs Against Value – Develop robust cost models that map directly to the business value you aim to generate. This ensures every pound spent has a purpose.
- Translate Costs into Business Metrics – Make AI spending understandable. Use granular consumption data to build high-level metrics that show ROI clearly.
- Ensure Data Compliance – All AI data use must align with your organisation’s privacy policies and ethical standards. Missteps can have costly legal and reputational consequences.
- Plan GPU Capacity in Advance – As AI demand grows, access to GPUs can become a bottleneck. Reserve compute power ahead of time to avoid performance delays.
- Strengthen Data Management Practices – Quality data is the foundation of AI. Good data management improves model performance and reduces operational waste.
- Prioritise Sustainability – Opt for energy-efficient models where possible. Reducing compute usage not only lowers costs—it reduces your environmental footprint too.
By integrating these practices, FinOps professionals can help ensure AI programmes deliver on their promises—without financial surprises.
Get the Full Guide
To explore the full strategy and practical frameworks behind AI cost optimisation, download The FinOps Practitioner’s Definitive Guide to AI Services today.
Additional Resources
- Gartner Research – When Should CIOs implement FinOps, ITFM, or Both?
- Thavron Solutions Learning Library
Want To Learn More? Let’s Start A Conversation.